Assistant Professor of Marketing
Ivey Business School, Western University
2021 - present
I completed my PhD in Marketing from the University of Michigan, Ross School of Business. My research interests lie in understanding and documenting the experiential consumption of digital products and media. I do this by implementing causal and/or interpretable machine learning methods on behavioral data.
In one project, I identify the relative importance of video modalities (across text, audio and images) in YouTube influencer videos that predict a change in viewer engagement. In addition, my analytical framework removes spurious associations between video features and engagement, thus shortlisting a subset of relationships which are more likely to have causal effects. In another project, I study the effect of four distinct ad delivery levers on ad avoidance and content avoidance behavior on streaming media. My findings show the contrasting effects of these levers and provide insights on design of ad delivery based on platform goals.
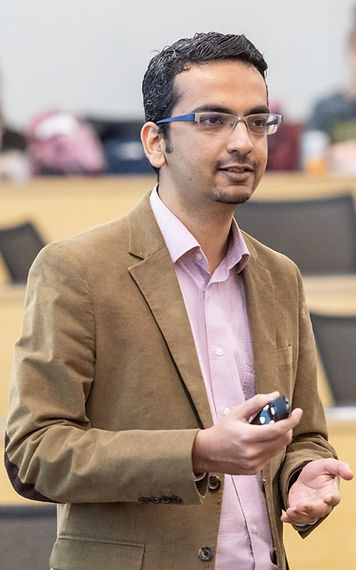